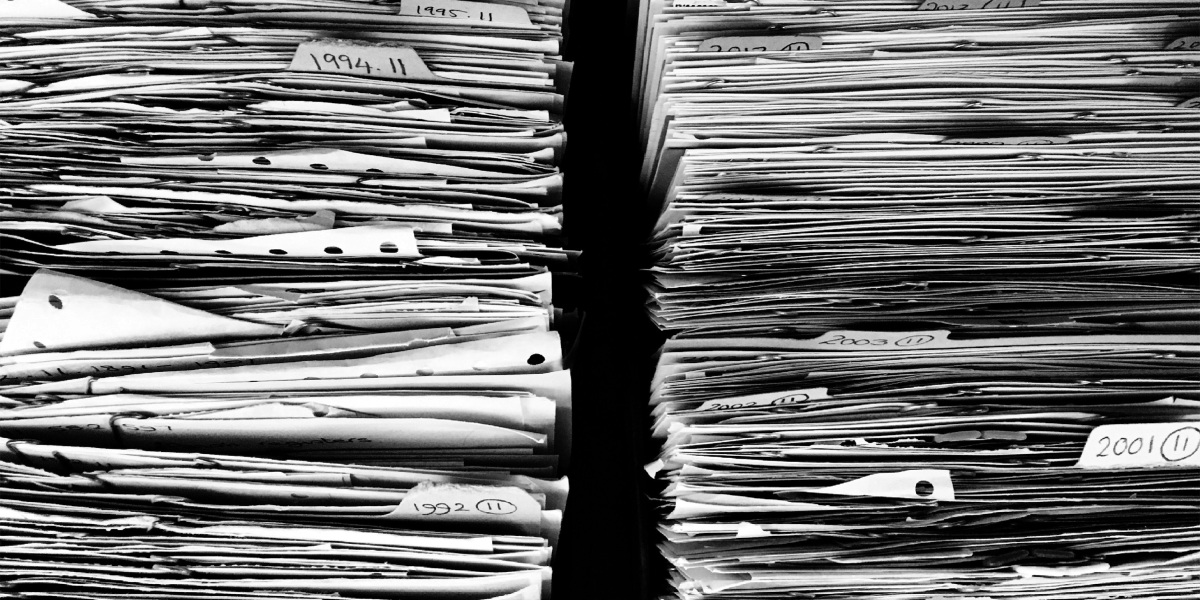
Now a team of Google researchers has published a proposal for a radical redesign that throws out the ranking approach and replaces it with a single large AI language model, such as BERT or GPT-3—or a future version of them. The idea is that instead of searching for information in a vast list of web pages, users would ask questions and have a language model trained on those pages answer them directly. The approach could change not only how search engines work, but what they do—and how we interact with them
Search engines have become faster and more accurate, even as the web has exploded in size. AI is now used to rank results, and Google uses BERT to understand search queries better. Yet beneath these tweaks, all mainstream search engines still work the same way they did 20 years ago: web pages are indexed by crawlers (software that reads the web nonstop and maintains a list of everything it finds), results that match a user’s query are gathered from this index, and the results are ranked.
“This index-retrieve-then-rank blueprint has withstood the test of time and has rarely been challenged or seriously rethought,” Donald Metzler and his colleagues at Google Research write.
The problem is that even the best search engines today still respond with a list of documents that include the information asked for, not with the information itself. Search engines are also not good at responding to queries that require answers drawn from multiple sources. It’s as if you asked your doctor for advice and received a list of articles to read instead of a straight answer.
Metzler and his colleagues are interested in a search engine that behaves like a human expert. It should produce answers in natural language, synthesized from more than one document, and back up its answers with references to supporting evidence, as Wikipedia articles aim to do.
Large language models get us part of the way there. Trained on most of the web and hundreds of books, GPT-3 draws information from multiple sources to answer questions in natural language. The problem is that it does not keep track of those sources and cannot provide evidence for its answers. There’s no way to tell if GPT-3 is parroting trustworthy information or disinformation—or simply spewing nonsense of its own making.
Metzler and his colleagues call language models dilettantes—“They are perceived to know a lot but their knowledge is skin deep.” The solution, they claim, is to build and train future BERTs and GPT-3s to retain records of where their words come from. No such models are yet able to do this, but it is possible in principle, and there is early work in that direction.
There have been decades of progress on different areas of search, from answering queries to summarizing documents to structuring information, says Ziqi Zhang at the University of Sheffield, UK, who studies information retrieval on the web. But none of these technologies overhauled search because they each address specific problems and are not generalizable. The exciting premise of this paper is that large language models are able to do all these things at the same time, he says.
Yet Zhang notes that language models do not perform well with technical or specialist subjects because there are fewer examples in the text they are trained on. “There are probably hundreds of times more data on e-commerce on the web than data about quantum mechanics,” he says. Language models today are also skewed toward English, which would leave non-English parts of the web underserved.
Still, Zhang welcomes the idea. “This has not been possible in the past, because large language models only took off recently,” he says. “If it works, it would transform our search experience.”