Part of the reason the company has focused its initial efforts on Canada is that the nation has large amounts of survey data in the public domain, including narrative field reports, timeworn geologic maps, geochemical data on drill hole samples, airborne magnetic and electromagnetic survey data, lidar readings, and satellite imagery spanning many decades of exploration.
“We have a system where we can ingest all this data and store it in standard formats, quality-control all of the data, make it searchable, and be able to programmatically access it,” Goldman says.
High-tech momentum
Once it has compiled all the available information for a site, KoBold’s team explores the data using machine learning. The company might, for instance, build a model to predict which parts of ore deposits have the highest concentrations of cobalt, or create a new geologic map of a region showing all the different rock types and fault structures. It can add new data to these models as it’s collected, allowing KoBold to adaptively change its exploration strategy “almost in real time,” Goldman says.
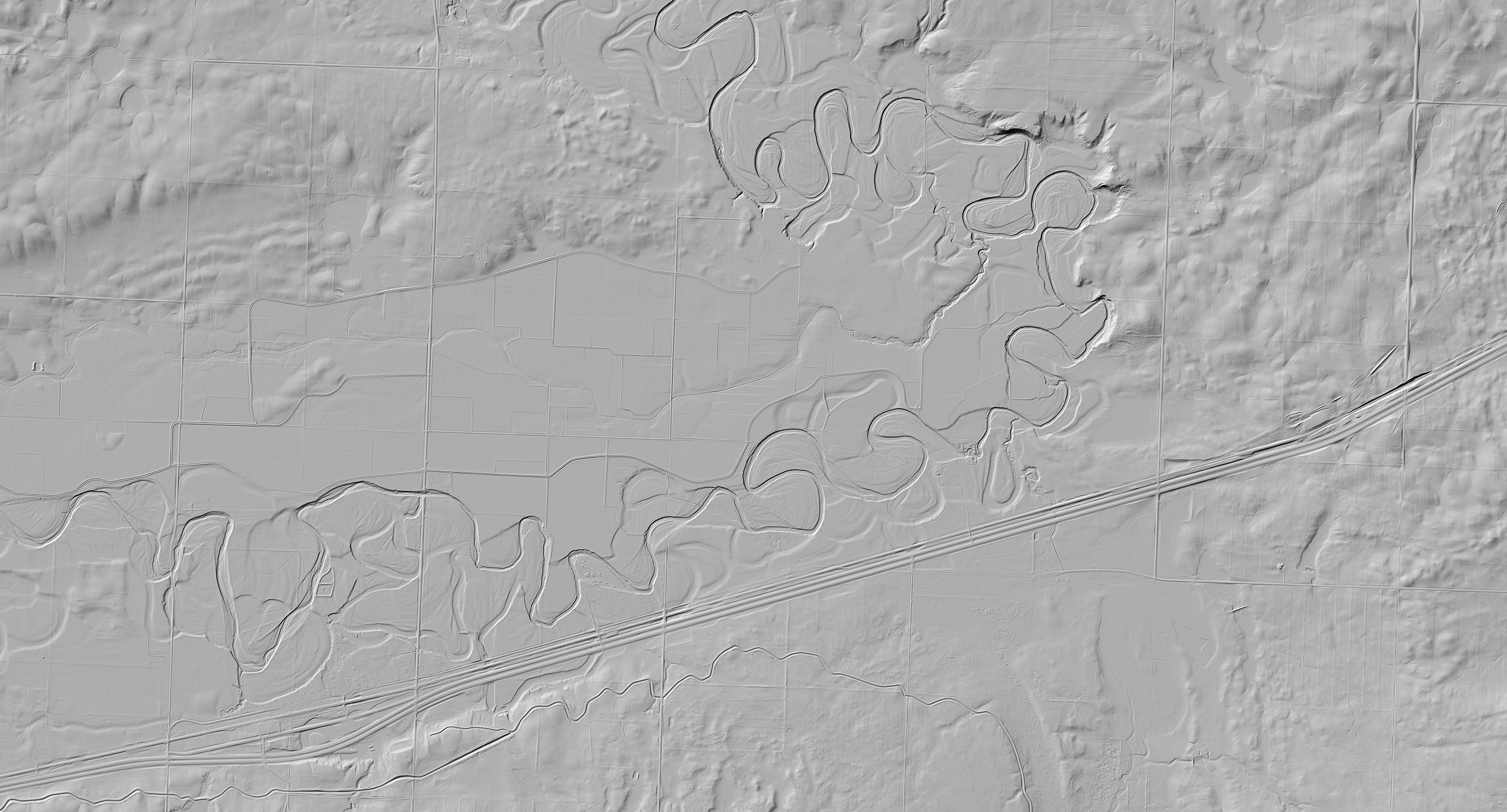
GOVERNMENT OF CANADA
KoBold has already used insights from machine-learning models to acquire its Canadian mining claims and develop its field programs. Its partnership with Stanford’s Center for Earth Resources Forecasting, under way since February, adds an additional layer of analytics to the mix in the form of an AI “decision agent” that can map out an entire exploration plan.
Stanford geoscientist Jef Caers, who is overseeing the collaboration, explains that this digital decision-maker quantifies the uncertainty in KoBold’s model results and then designs a data collection plan to sequentially reduce that uncertainty. Like a chess player trying to win a game in as few moves as possible, the AI will aim to help KoBold reach a decision about a prospect with minimal wasted effort—whether that decision is to drill in a particular spot or walk away.