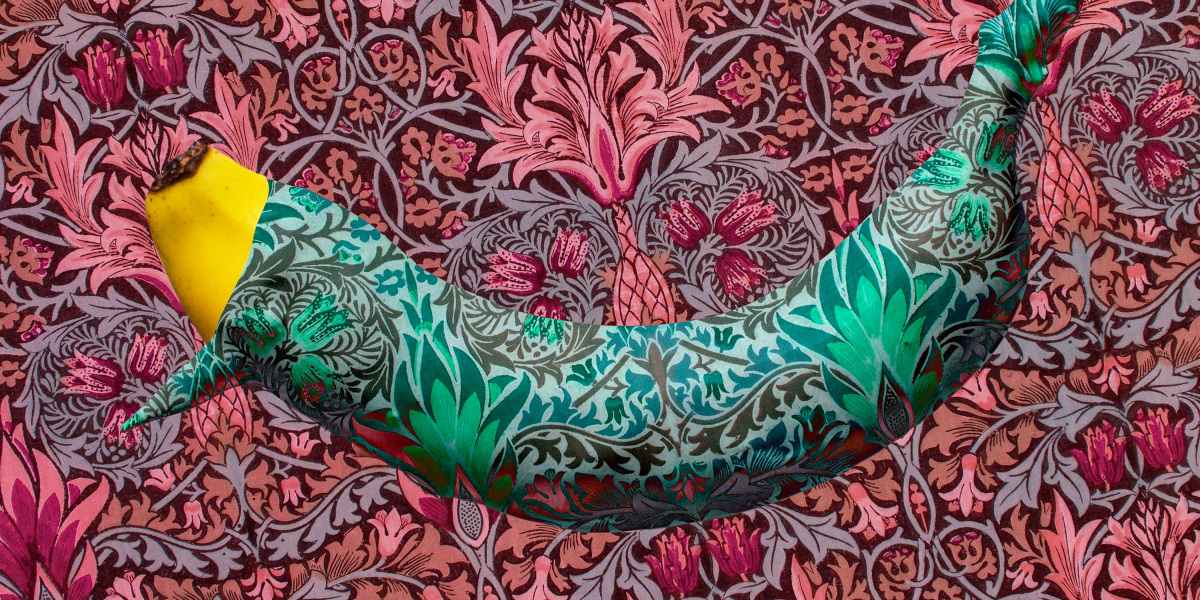
The results point to one of the most challenging aspects of AI-based hate-speech detection today: Moderate too little and you fail to solve the problem; moderate too much and you could censor the kind of language that marginalized groups use to empower and defend themselves: “All of a sudden you would be penalizing those very communities that are most often targeted by hate in the first place,” says Paul Röttger, a PhD candidate at the Oxford Internet Institute and co-author of the paper.
Lucy Vasserman, Jigsaw’s lead software engineer, says Perspective overcomes these limitations by relying on human moderators to make the final decision. But this process isn’t scalable for larger platforms. Jigsaw is now working on developing a feature that would reprioritize posts and comments based on Perspective’s uncertainty—automatically removing content it’s sure is hateful and flagging up borderline content to humans.
What’s exciting about the new study, she says, is it provides a fine-grained way to evaluate the state of the art. “A lot of the things that are highlighted in this paper, such as reclaimed words being a challenge for these models—that’s something that has been known in the industry but is really hard to quantify,” she says. Jigsaw is now using HateCheck to better understand the differences between its models and where they need to improve.
Academics are excited by the research as well. “This paper gives us a nice clean resource for evaluating industry systems,” says Maarten Sap, a language AI researcher at the University of Washington, which “allows for companies and users to ask for improvement.”
Thomas Davidson, an assistant professor of sociology at Rutgers University, agrees. The limitations of language models and the messiness of language mean there will always be trade-offs between under- and over-identifying hate speech, he says. “The HateCheck dataset helps to make these trade-offs visible,” he adds.